
This blog was written by Patrick Miller & the Flywheel Data Science Team
Flywheel has led the industry in evaluating programmatic efficiency- building custom solutions such as a site filtering algorithm to evaluate Amazon DSP sites for high-value metrics and a frequency capping model that determines optimal frequency across DSP sites. Here’s what inspired us to build ahead of the industry:
A programmatic ecosystem focused on scale and not performance
For years, publishers have crammed their sites and apps with so many ads that users ignore them. Flywheel decided to dive into this issue by looking at a variety of metrics at the site/app level using Amazon Marketing Cloud to determine which sites were effective at driving retail performance for our clients - we knew it wouldn’t be all of them. This led to the development of our own site filtering algorithm, a solution which focuses delivery on sites that are driving true performance. To us, performance means closed-loop retail metrics and not high CTR, impressions or other metrics that can be false positives. For more on our site filtering solution, check out this blog from earlier this year.
These types of publishers, inclusive of MFAs, came into the spotlight recently following a report released by the ANA around programmatic supply chain transparency. The report validated what we always knew to be true. There’s a lot of wasted spend in the programmatic space, and it has been perpetuated by advantageous publishers looking for massive scale.
But as the saying goes, it takes two to tango. On the other side of the table, you also have advertisers chasing cheap CPMs. Without a closed loop and thus inability to gauge efficacy deterministically, brands thought they could chase low CPMs and still achieve reach objectives. While mathematically true, it was practically false, i.e. the CPMs were low for a reason….junk inventory (read more here). The next big driver of performance we decided to focus on was frequency management. Let’s talk about frequency capping.
Frequency capping is defined as an advertising tactic that limits how often an ad is shown to the same person during a specific time period. Some advertisers are okay bumping up frequency caps or even removing them entirely in exchange for more ad impressions. And the inverse is also sometimes true - a report by Adalytics cited a few examples where advertisers were paying eCPMs north of $1,000 to reach a single customer on a single website. We have low conviction on what the ideal frequency should be and high conviction on what the ideal outcome should be: drive incremental, long-term revenue for brands and use deterministic measurement to prove out investment and product unit economics. To look at this issue in more depth, we decided to use Amazon Marketing Cloud to evaluate DSP ad spend for one of our advertisers. Our goal was to see at what ad frequency did the brand reach a specific % of their overall sales for the month?

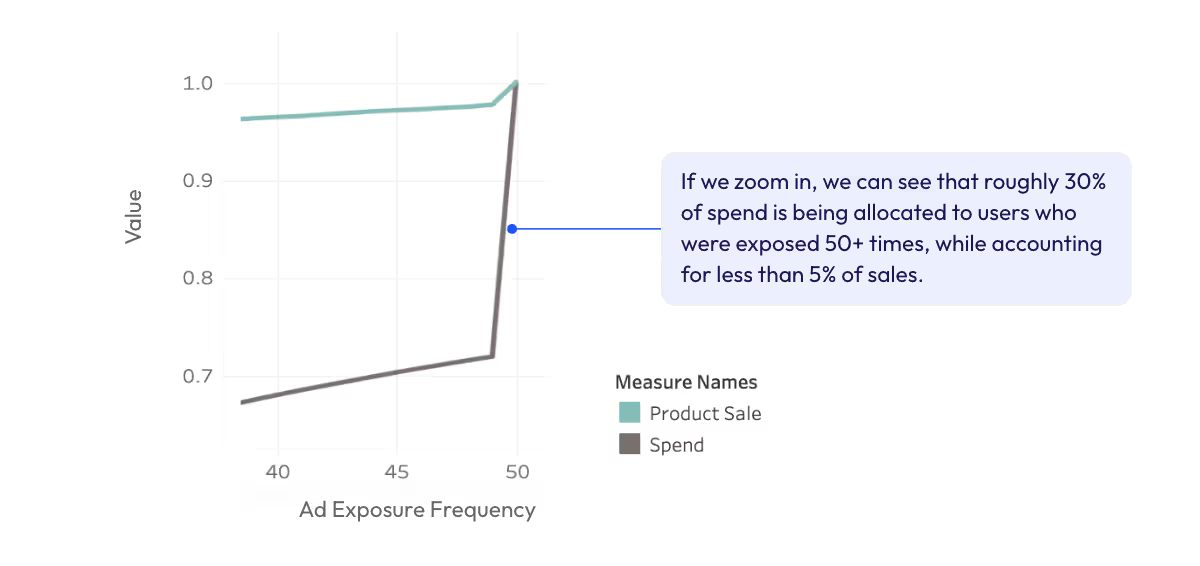
We found that the advertiser had reached 90% of their monthly sales on users that were exposed to ads 18 or fewer times. This means that users exposed to ads beyond that frequency 18-49 times only accounted for 10% of monthly sales, clearly indicating a dropoff in purchase intent, and showing a diminishing return in the additional impressions delivered to those users.
Enforcing a flexible frequency cap to reallocate spend
Knowing that many consumers are likely being over-exposed to ads within the DSP, we wanted to figure out a way to identify the optimal frequency cap to effectively drive a user to take an action, such as a detail page view or purchase. To solve for this, our team developed a frequency capping model that evaluates spend across DSP tactics to see when certain metrics become saturated (like the example above) and start to see diminishing returns. Once the threshold is defined, our model can then generate a recommended cap at a weekly, monthly, or quarterly range. With this frequency cap in place, an audience can then be made to exclude users shown that number of ads in the past X days, and it can be updated continuously to get new recommendations for the frequency cap.
For one of our clients, we implemented the frequency cap model using 50 impressions per user per month across the brand as the threshold. With the frequency cap in place, most campaigns continued to deliver at 100%, which was a main concern of the advertiser. Some lower funnel-focused campaigns did see a decrease in spend up to 50%, however, this had no impact on sales.
.avif)
When looking at the variance in certain ad metrics, we saw noticeable improvements with frequency caps in place. Detail page view rate increased 45%, NTB purchase rate increased 66%, and cost per NTB decreased by 23%, illustrating the immediate impact the model had on performance. Similar to our site filtering algorithm, we found that CPMs were actually higher when utilizing the frequency capping model. This is to be expected when implementing a more strict frequency cap, but a fair tradeoff when you consider the lift in performance for the metrics highly correlated with increased GMV.
Customized models for each brand
The frequency cap model explained above and the filtering algorithm explained in the previous post are tuned to each brand and are unique to each brand. Every brand and their objectives are unique and by focusing on the outcomes they want to achieve we can ignore much of the noise of the programmatic industry and focus on deterministic value creation. And while we would love the entire internet to be deterministically measurable, it’s not and will never be possible.
For a quick visual of these solutions, check out our videe series: First Look: ADSP Site Frequency & Optimization
Ready to grow your business?
Let’s discuss the best approach to meet your brand’s specific needs.
Let's connect